Those of you who have experienced online dating will know quality connections are not easy to find! When a ‘spark” appears there is usually a flurry of messages, one more intimate than the last, as each person progressively disclosures a little bit more about themselves. Some people know the art of progressive disclosure so well, that they can quickly create a connection that might take months or weeks in the real world!
If you have used a Dating Apps (even a sneak peek), you will also know that Dating Apps use their own type of progressive disclosure (aka progressive onboarding), to make sure the App becomes extremely habitual.
So what can Dating Apps teach us about the art of seducing users??
Progressive Onboarding Tip 1: Create a seducing first-time experience.
When you download a Dating App like OKCupid or Bumble, the first actions are to login in with your Facebook account, upload a photo and start swiping. These Apps want to bring you to the buzz of “a match” as quickly as possible. There are no busy onboarding carousels describing the product benefits or how to use the App, they know, this all gets in the way of the first time user experience.
Dating Apps don’t want to overwhelm users with all features and possibilities and prefer to give users “instant gratification”. They make it as easy as possible for the user start swiping, then use progressive disclosure to show uses the more advanced features of the App at a later stage.
This is how Bumble does it – download, login, swipe!
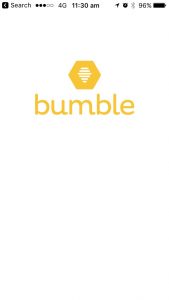
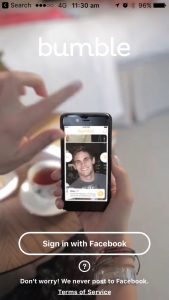
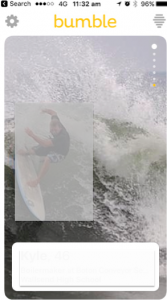
Even after the first the swipe, you have no idea about the features of the App, what is free or isn’t or how to customise searches or experience. That’s all a mystery at this stage and they just want you to play!
Progressive Onboarding Tip 2: Only ask users to pay after they have received value
Progressive disclosure is the best way to show people the basics first. After they understand the core value of the App and are hooked, show them the higher value paid features. Your chance of getting a user to upgrade to paid features is much higher after they have had an awesome first-time experience.
This is how Bumble asks people to pay for more valuable features.
The Bumble App, puts women in control, as only women can initiate a conversation with a match. The men cannot start a conversation with a woman, they can only show interest, by liking. After the female user has achieved some matches and visits her queue, it is then disclosed that she can upgrade her subscription to see everyone that has liked her.
Very clever and so much cleverer than explaining the paid features upfront, which is a less effective practice that many less mature Apps use.
This Bumble use case is a great example of disclosing real-time user education about paid features when the user is ready and motivated to hear about them.
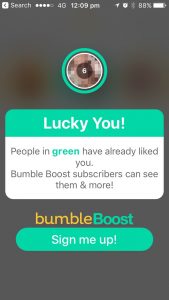
Progressive Onboarding Tip 3: Let their users loose to learn about the App
One way to think about progressive disclosure is to only show the information that is relevant to the task that a user wants to focus on. When you think about onboarding in this way, it puts the onboarding journey in the hands of the user rather than the App developers. We call this self-paced onboarding, where the users learn about the App by experiencing the Ap at their own pace.
Let’s look at another great seducer – OKCupid, and how they get feature activation by showing users how to be more productive in the App.
In this case, a user after experiencing the App for some time is shown the Search Filter feature. OKCupid has decided to not show this important feature to users in the early stages of their journey. They have opted to delay this piece of user education until the user has been engaged and active for some time, and enjoying the experience of finding matches and chatting with potential dates.
Segmenting users “who have not used the search function” and presenting a tip to show users where to find the search function, is a great way to provide contextual education. It is simple, relevant and is valuable as it focuses the user on the benefit – save time and find better quality matches.
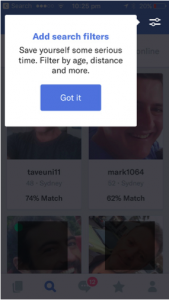
Progressive Onboarding Tip 4: Help users understand the features they are paying for!
In the online dating world, everything happens fast. So when a user agrees to pay for access to subscriber features, it’s important they understand what they have access to and how to use the features. Reminding users of the value of features is a strategy used by OKCupid as a way to demonstrate the exclusive benefits of being a subscriber.
For example, OKCupid paid users, have the benefit of secretly viewing profiles. This means they have the option to let someone know that they showed interest by visiting their page. In this example, education is triggered and targeted to a segment of users – “paid users” who have never performed the “reveal my visit” option.
Triggering a tip in real-time is so much effective and relevant compared to education outside of the App via email or push notifications that users rarely read. The “Got it” button, also means the OKCupid can measure the effectiveness of the education, and attribute feature usage to an education campaign.
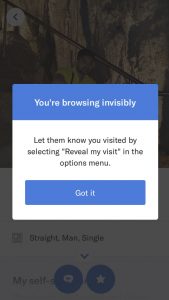
How can Contextual improve your Apps progressive onboarding?
In the dating world, progressively disclosing interesting information is so much more attractive than doing a download of your history. The same goes for user onboarding, and the leading Dating Apps show us the art of seduction – giving the user an instant experience of a match, then progressively showing users how to improve their experience over time.
The Contextual platform makes it super easy for all Apps to have this superpower. With Contextual, all Apps can create tips, tours and modals to progressively disclose important features to a user without getting in the way of the user’s experience.
This is all done without code or waiting for an App release.
Make your App more attractive and:
– Push feature usage to segments without code
– Take the guesswork out of feature engagement
– Avoid having to code for the tip to disappear once the App is open or the user has engaged
– Get data in and out of the platform with a REST/JSON API and target the right users in real time